Published on the 14/09/2017 | Written by Donovan Jackson
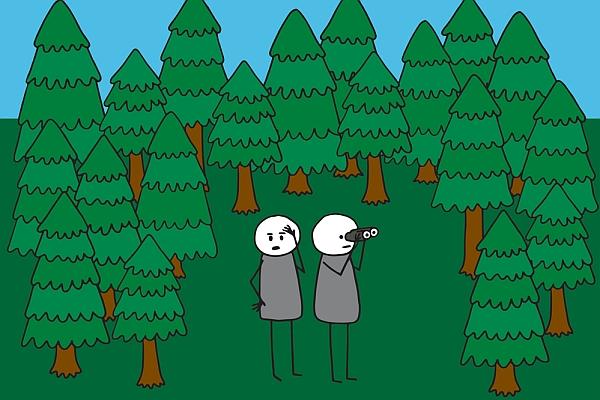
Structured, unstructured and lots of it – but does more data translate more sense?…
Analytics is kind of a big deal right now, particularly since it is among the steps taken on the path to artificial intelligence. But the step which comes before analytics is data and the orthodoxy is the more data you have, the better the analytics you could have (we’ll take a closer look at that hypothesis shortly). And while there is no shortage of data, the trouble has always been grabbing hold of it and then making it useful. Which is where Cloudera and other vendors in the ecosystem which has popped up around Apache Hadoop come into the picture. There’s even a term for the types of issues these vendors (and their partners) set out to solve: Hadoopable problems. These include such things as risk modelling and customer churn analysis. Those problems, said Mark Micallef, Cloudera Asia Pacific Japan VP in a recent chat with iStart, tend to be found in data-rich industries: telcos, financial services, ‘and of course government’, IoT and manufacturing. “That’s where we see growth coming from; notably, A/NZ is ahead of some of the other APJ regions in its adoption of big data, ahead of even Japan, with around 20 percent of our customers running Cloudera Enterprise in public cloud infrastructure.” The commonality in these industries is that they have very large data sets on the one hand. “In a lot of these industries, the sheer volume of data has increased to such an extent that it is cost-prohibitive to store in the traditional data warehouse,” Micallef said. On the other, they also have a ton of unstructured data which doesn’t quite slot into the traditional method of storing information – relational databases. Data like, ye gods, Twitter and Facebook feeds, call centre conversations and internet chatter. “For data intensive activities, organisations need to build a platform into which this data can be offloaded and where they can store immense volumes with a distributed structure. And while that can address immediate problems, it also establishes a resource which they can use to see what else they can do with it by applying machine learning or advanced analytics for more value longer term.” Wood or trees? Hadoopability comes where there’s value in analysing all sorts of data, including, apparently, the likes and gripes from Twitter or Facebook. In any case, Micallef said this is a clear example of accessing and using unstructured data; “The proportion of unstructured data has grown to 60 percent. And unstructured data is inherently relevant to most businesses.” The analysis gaps “While we need to ask what we are missing if we are not looking at unstructured data, we first need to realise that just 50 percent of structured data is being analysed. And just one percent of unstructured is being analysed,” Micallef said. “And as we know, data is worthless unless you do something with it.” The analytics vendors and associated hangers-on would have you believe that therein lies enormous advantage. After all, if you’re getting results from analysing that 50 percent, just imagine what wonders lie in the remaining cohort. And what about the 99 percent of unstructured data? Surely rich insights are to be found. Of course, such an argument holds water. However, there is an alternate view, one of which is quite simply the concept of using a representative sample, which has since time immemorial provided the ability to extrapolate insights from small data sets to large ones with some degree of confidence. The question of course should be framed as ‘does more data deliver better insights’? To a relatively obscure source we turn: KDNuggets goes into some detail about why ‘more data does not always help’ (in the context of whether more data or better algorithms are necessary). A Harvard Business Review article, albeit from the dark ages of 2013, explains nicely why cranking open massive data sets doesn’t necessarily translate into much more than having more data sets to work with. Trash or treasure? Asked if unstructured data really is ‘all that’, or instead just a load of old cobbler’s awls, Micallef said a good deal of the Hadoop ecosystem is dedicated to cleansing it and making it more meaningful. For example, that’s what Talend does; it focuses on getting the data in from all the different sources, then using effective processing to make sure it is cleansed and meaningful,” he explained. Just how much meaningful information remains after so fisking a teenager’s Facebook blather is perhaps up for debate. Finally, throughout the interview with iStart, Micallef mentioned the so-last-year buzz term ‘big data’ just once. Asked why that was, he said ‘big data’ is still very pertinent and some customers still call it that (although Doug Cutting, Hadoop inventor, reckons ‘it’s just data‘). “However, in some cases this is a term that has not fully captured the problems that we are trying to solve. The traditional use cases of data warehouses to offload and store huge volumes of data led to the term and it is where customers are heading. A lot have done phase one, which is getting data into the [Hadoop] platform cost effectively. Where we’re heading now is they are readying up on how to use machine learning, whether supervised or unsupervised, to get real value from the platform.” … It’s time for AI to go from low impact to big bang… Mercy Radiology’s $200k cash bonus from RPA… New research suggests the human touch might be the solution to the excesses of the big data deluge… Data lakes, traceability and partnering key… Facial recognition at airports – is its beauty skin-deep, or ugly all the way to the bone?…
Just one ‘Hadoopable problem’ to which Micallef drew attention is customer churn for telcos. It’s an age old problem and when poorly understood can undermine the most successful businesses. “It is a very typical use case; the customer service rep wants a 360-degree view of customers and the business is looking to reduce churn. They need the ability to use data to know ahead of time when someone is more likely to drop their subscription. By knowing the behaviour of customers, the rep – often with the help of automated marketing systems – can intervene with an appropriate deal before the subscription is cancelled.”
With the banter around analytics reaching something of a crescendo, one might imagine that the raw material for the stuff is constantly receiving the attention of a fine-toothed comb. One would imagine wrong.
There’s one more factor worth considering: perhaps all that unstructured data is just so much chatter and nonsense. Those who write for a living, for example, know that a directly transcribed interview (or call centre discussion, for that matter) makes little sense. Most of the words spoken are ‘fluff’ and don’t directly communicate anything. Twitter and Facebook feeds are noted for the potential for distortion of meaning.FURTHER READING
Stuff taking AI mainstream
Switched on CEO: Dr McCann builds a bionic business
Ethics and algorithms: Can machine learning ever be moral?
Switched on CIO: Andrew Goodin goes for gold with Zespri
Qantas/Sydney international airport embrace biometrics