Published on the 10/08/2018 | Written by Heather Wright
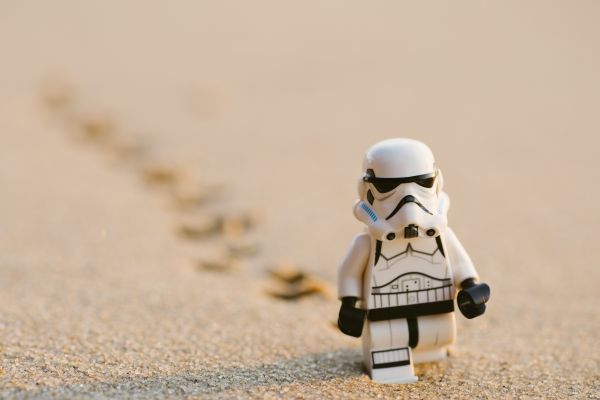
Trust is a must with all technology, but will AI legislation stymie innovation?…
Governments around the world are scrambling to put together legislation, guidelines and codes of ethics, around AI as companies – and countries – expand their efforts to compete in the growth market.
The challenge should not be underestimated given that many legislatures are still grappling with digitisation, let alone automation.
While much of the attention so far has been around preventing weaponisation of AI and killer robots, and the regulatory issues around autonomous cars and drones, the focus is moving to business use of AI and the hidden ‘black box’ algorithms that drive it.
“It is unfortunate that some of the most powerful among these cognitive tools are also the most opaque.”
It is, after all, a big market. Gartner has forecast that global business value from AI will hit US$1.2 trillion this year, an increase of 70 percent on last year largely driven by decision support/augmentation tools, which allow data mining and pattern recognition enabling algorithms to work directly with information.
At the same time, governments, including Canada, China, France, Japan and the United Kingdom, have all launched initiatives to speed their country’s AI development. In Australia, the federal government’s 2018-19 budget earmarked $29.9 million over four years to grow AI capabilities in sectors including health, agriculture, energy, mining and cybersecurity, and develop an ethics framework and a standards framework. The New Zealand government is also eyeing up AI, with New Zealand’s minister for government digital services Clare Curran noting in May that the country was lagging behind comparable countries in its work on AI and ethical issues, and promising to ‘move quickly’ on both. A project looking at NZ government use of algorithms is due to be completed this month.
But the ethical issues around AI, data privacy, algorithms and legislation are proving complex – and fraught with concerns over the stymieing of innovation.
Among those leading the way with legislation is the EU. The GDPR, which came into effect in May requires companies to provide ‘data subjects’ with ‘meaningful information about the logic involved in the decision’, according to the European Commission, which has set up a group to draw up proposed guidelines for AI ethics.
“Without direct human intervention and control from outside, smart systems today conduct dialogues with customers in online call-centres, steer robot hands to pick and manipulate objects accurately and incessantly, buy and sell stock at large quantities in milliseconds, direct cars to swerve or brake and prevent a collision, classify persons and their behaviour or impose fines,” the European Group on Ethics (EGE) says.
But, the group notes, ‘it is unfortunate that some of the most powerful among these cognitive tools are also the most opaque’.
“Their actions are no longer programmed by humans in a linear manner. Google Brain develops AI that allegedly builds AI better and faster than humans can. AlphaZero can bootstrap itself in four hours from completely ignorant about the rules of chess, to world champion level.”
The EGE says it is near impossible to understand exactly how AI is improving its own algorithms. “Deep learning and so-called ‘generative adversarial network approaches’ enable machines to ‘teach’ themselves new strategies and look for new evidence to analyse. In this sense, their actions are often no longer intelligible, and no longer open to scrutiny by humans.
It is impossible to establish how they accomplish their results beyond the initial algorithms, the EGE says, and their performance is also based on data used during the learning process and that may no longer be available.
“Thus biases and errors that they have been presented in the past become engrained into the system.”
But the EU’s approach isn’t supported by everyone, with the Center for Data Innovation – a US research institute – saying it would be a mistake for the United States and other nations wanting success in the digital economy to opt for ‘this precautionary-principle path’.
In a recent report, Joshua New and Daniel Castro from the Center for Data Innovation, say risks such as racial bias and advertising algorithms promoting high paying jobs to men more than women, are ‘often overstated, as advocates incorrectly assume market forces would not prevent early errors or flawed systems from reaching widespread deployment’.
The pair argue that heavy-handed legislation such as prohibiting the use of algorithms where companies cannot explain their decision making (algorithmic explainability) or mandating businesses disclose source code (algorithmic transparency), will limit innovation, reducing a company’s incentive to develop new algorithms as it opens the doors for competitors to copy, while failing to prevent consumer harm.
Instead, they advocate algorithmic accountability as a light-touch regulatory approach, whereby algorithmic systems use a range of controls to ensure the company using it can verify it acts as intended, and identify and rectify harmful outcomes.
“Adopting this framework would both promote the vast benefits of algorithmic decision-making and minimise harmful outcomes, while also ensuring laws that apply to human decisions can be effectively applied to algorithmic decisions,” they say.
No doubt, it’s a complex area. It may just need an algorithm or two to resolve it.