Published on the 31/10/2023 | Written by Heather Wright
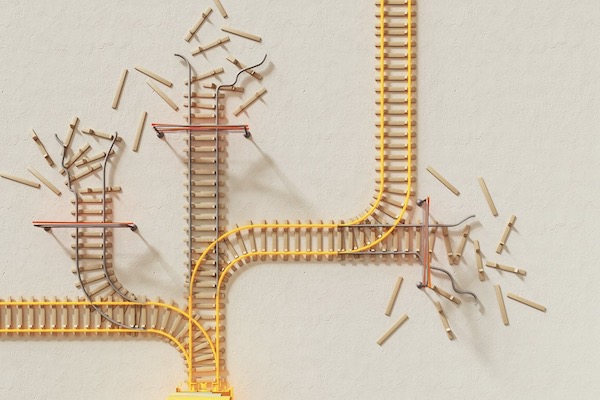
Think of your generative AI platform as a four-layer cake…
Gartner has urged organisations to use enterprise data model APIs to protect privacy of data when it comes to deploying generative AI in enterprise situations.
At the recent Gartner IT Symposium, Arun Chandrasekaran, Gartner distinguished vice president, analyst, noted that there are plenty of ‘non-trivial’ challenges to generative AI for enterprises.
“We need to build a platform agnostic of models so we have the ability to swap models and reduce vendor lock-in.”
In a standing room only presentation Xpo – which saw organisers rushing to open additional space for overflowing attendees clamouring to hear about generative AI – Chandrasekaran laid out the enterprise implications of ChatGPT and how CIOs could avoid some of the avoidable mistakes as they harness the technology.
Key among the risks is loss of intellectual property, either through data being used as an input to a model potentially becoming a public output of the model, and loss of data as companies move data to external environments to do prompt engineering or fine-tuning of models.
The black box nature of models and their lack of training data attribution, and ability to hallucinate are also challenging organisations, Chandrasekaran says.
Those risks, however, need to be balance against potential benefits including near-term workforce productivity gains – which he says are already being seen in many different functions including customer service, marketing and comms, software engineering and HR – and the versatility of the models which are capable of being deployed across different domains.
Longer term is the promise of democratisation of knowledge, putting both organisational and external knowledge at the fingertips of every worker within a company and, even more importantly, democratisation of skills.
“Are the risks or generative AI real? Yes,” he says. “Can we completely eliminate the risk of generative AI today? No. Can we mitigate most of these risks through training, technology and processes? The answer is absolutely yes.”
Gartner data shows that close to 20 percent of organisations are in some stage of generative AI adoption. Of those, slightly less than five percent have workloads in production, with the remainder piloting. Seventy percent of companies are in ‘active exploration’, Gartner says, with only 10 percent saying they’re currently doing nothing about the technology
That begs the question of what organisations are actually doing with generative AI?
Chandrasekaran says the most common use cases are in the natural language domain, such as content generation and summarising lengthy documents – something Chandrasekaran says there has been an acceleration of in recent months thanks to increasing context window sizes enabling more words to be processed in a single prompt.
But its enterprise search which Chandrasekaran says is a more strategic use case within the natural language processing arena. That’s enabling organisations to reimagine search to be more interactive and semantic oriented, searching on similarity, rather than just keywords.
“Every CIO we talk to says they want to deploy a private ChatGPT within their firewall because they want a personalised Q&A system that really taps into [the company] knowledge and want to put it in front of their knowledge workers.”
Computer vision examples are also coming to the fore, including with computer vision automation in industries such as manufacturing and retail, where organisations are combining the power of large language models and computer vision to add meta data to images for example.
But risk mitigation remains a critical aspect of operationalising generative AI models. That’s where Chandrasekaran recommended organisations ‘almost always’ use enterprise data model APIs, for example using ChatGPT Enterprise, the ChatGPT API or the GPT 3.5 model available in Azure cloud because ‘it comes with a guarantee that we won’t use your data to train the model’.
Rag (retrieval augmented generation) architecture and fine tuning can reduce model hallucinations through grounding models with your own enterprise data.
Equally, the Rag architecture, along with explainability tool kits, can help make models explainable and add a sense of referenceability, demystifying the black box nature of the models, he says, and MLOps observability tools that observe anomalous behaviour of models can prevent misuse of the model.
There is, of course, also the human aspect, with Chandrasekaran calling on companies to upskill their teams with skills such as prompt engineering and provide clear career paths and even emotional assistance.
Chandrasekaran urged companies to create an environment where people can rapidly experiment and build prototypes, building sandbox environments for creators to experiment with the technology.
Hackathons are an increasingly popular way to institutionalise and gamify the experience of developing applications using generative AI models, he noted.
For companies who have decided to build a generative AI application, Chandrasekaran noted that he thinks of the platforms as a four-layer cake.
The bottom layer is the infrastructure layer. Generative AI requires lots of compute power, particularly GPUs – currently in short supply – to train and finetune models.
Layer two is the models themselves, coming in a variety of categories, including the massively pretrained foundation models like GPT 4; domain models, which are typically mid-sized or smaller models which are very case specific, and verticalised domain models trained on data specific to a particular area, such as health or financial services. Model hubs – essentially marketplaces where open-source models are curated and available for developers – are also playing an important role. Hugging Face is one such model hub.
The third layer of the platform is the tools to bring the models to production, such as tools for finetuning models, scaling and doing model validation, ensuring responsible, ethical deployment and connecting the models – an area Chandrasekaran notes will be important for many companies as they seek to connect multiple models together.
Plenty of MLOps for GenAI tools already exist across the categories, with offerings rapidly swelling.
The final layer is the exploding market of horizontal and vertical apps.
“The platform approach you take is particularly important because the models we are using today may not be the models you will be using a year from today because of how fast this ecosystem is moving.
“We need to have the ability to build a platform that is agnostic of models so we have the ability to swap these models and reduce vendor lock-in to the extent possible,” he warned.
“Carpe diem – seize the day. Identify what generative Ai means for your organisation in terms of looking at benefits, risks opportunity and timeline for deployment. Make responsible AI front and centre of your generative AI initiative. Make sure you are upskilling employees on appropriate machine human interaction. Make sure you have a tiger team that’s really staying abreast of fast-moving market developments. And finally, help your employees through this with upskilling and career mapping.
“This is not futuristic. It is happening in front of us.”