Published on the 10/05/2023 | Written by Heather Wright
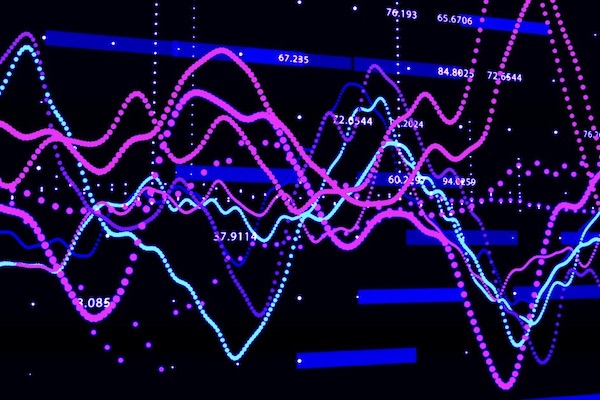
And helps identify true ‘peers’
University of Auckland Business School academic Paul Geertsema is urging New Zealand and Australian companies to look more closely at machine learning and what it can do for local businesses.
His comments come after he and colleague Helen Lu successfully trained machine learning algorithms to evaluate the actual value of companies, based on profitability, efficiency, growth and risk, among other metrics, and to help identify true ‘peer’ companies.
“Machine learning algorithms provided more accurate valuations of stocks than traditional methods.”
In a paper published in the Journal of Accounting Research, Lu and Geertsema show that the machine learning algorithms can provide more accurate valuations of stocks than traditional methods such as discounted cashflow analysis or the multiples approach/relative valuation.
Geertsema says the algorithm was trained on thousands of data points of US companies, where it could see both the accounting data and the actual value of the company as it traded on the exchange. It has since been trained on Australian and New Zealand data as well.
Lu says determining the value of a company involves many subjective choices and therefore its stock prices can be influenced by human bias, making the machine learning methodology ‘game-changing’.
While it could be used for companies preparing to list, Geertsema told iStart it could also prove a valuable tool for those already listed.
“What we found is that the valuations that the machine learning algorithm came up with in our study almost had a characteristic of being a fundamental value in the sense that over the next month the actual market value moved towards the machine learned valuation,” he says.
The paper also showed the model could be used to design a profitable trading strategy, with stocks identified by the algorithm as being undervalued tending to rise in price, giving investors the opportunity to profit without taking on risks. If implemented, it could return a ‘moderate’ profit of around four to five percent per year.
The objective for Lu and Geertsema however, wasn’t to make money with trading strategies, he says. Instead, it was to test whether the machine learned valuation could be a proxy for fundamental value, particularly since it was trained using only fundamental accounting data – the actual account information reflecting the performance of a company.
“If the market price is a lot higher than the fundamental value, you’d expect it to come down a little bit in the next month. That gap between the fundamental price and the valuation should give you information about the stock in the next month, and we found it did.
“Machine learned valuation acts as a proxy for the fundamental value of the firm and occasionally the market might deviate from that but then tends to revert back to more of the fundamentals.”
While similar approaches are already being used by those in the fund management industry, where they are highly guarded, Geertsema says the machine learning algorithm has some potentially significant ramifications, providing a reality check for valuations. The machine learning approach, which he believes would be a good complement to traditional methods, is also much cheaper than discounted cash flow analysis which can cost thousands of dollars and is time intensive.
Lu and Geertsema also developed machine learning algorithms to help companies identify peer firms when traditional methods fall short – something especially useful in countries like New Zealand where finding an obvious peer can be a struggle.
“What we show in our paper is that whereas historically people have done that almost exclusively on the basis of industry, that doesn’t necessarily need to be the case,” Geertsema says.
“You can find firms that are comparable even though they might be in a different industry – they are comparable in terms of how the machine learning algorithm looks at valuing companies.”
The peer companies can then be used to do a relative valuation.
Despite the success of the model, and approaches from companies wanting to collaborate on the research, Geertsema says there are no plans for commercialisation currently.
“The truth is that the large companies – the investment banks and large asset managers – they can do this in house and don’t need a third party to do it for them. Which means if you were to commercialise it you would be looking at the smaller players. That’s not to say it’s not attractive, but it’s not attractive for us right now.”
The success, however, does highlight the potential for machine learning for businesses, he says.
“These machine learning tools are now mature. They’re quite powerful. They’re not a magic bullet and can’t do the impossible but they can be very useful when applied in the right settings.
“Yes, you do need to learn to use them but that is not insurmountable.
“The internet was also at one point new and we learned how to use it, and I think machine learning now is the new internet. It is the new tool everyone will need to become at least to some extent familiar with and businesses that are able to use these machine learning technologies will have a definite advantage in terms of making better decisions, better understanding the data they work with, and being in general just a little bit more competitive.”