Published on the 07/11/2024 | Written by Heather Wright
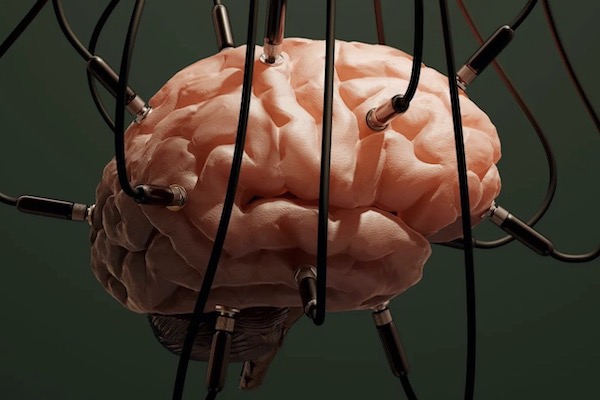
Know thy strengths – and weaknesses…
IT leaders need to challenge their vendors, IT and business teams and call them out if they want to use generative AI for the wrong problem – and take the money being offered amid the excitement around the technology and channel it instead into solutions ‘that are really going to work’.
Bern Elliot, vice president and distinguished analyst with Gartner Research, says since 2022 many of us have been focusing on when to use GenAI, but we really need to start thinking about when not to use the technology.
“AI is a toolbox and GenAI is just one of the tools in that toolbox.”
A Gartner survey last year showed generative AI was the most popular AI technique in use among respondents inching ahead of other techniques including machine learning (28 percent), NLP (27 percent), optimisation techniques (25 percent), graph techniques (22 percent) and rule-based systems (22 percent) to claim top spot at 29 percent in its first every showing.
But Elliot says its time organisations got real about where generative AI is actually useful, and where other techniques are the better option, rather than being swept away by ‘the biggest hype Gartner has ever experienced – two or three times higher than any other hype we have seen’.
He noted many solutions already in production where AI has been ‘really successful’, including supply chain optimisation, sales forecasting, customer churn prediction, personalised shopping recommendations, predictive maintenance, autonomous systems and robots, analysing for damage for claims processing and marketing allocation decisions.
“The key words here are optimisation forecasting prediction, personalised recommendations, maintenance, autonomous systems, analysis, decisions. These are the fundamental AI capabilities that are behind.
“But what all these well-established solutions have in common is that GenAI is not the primary AI method.”
Generative AI is strong at content generation, knowledge discovery and conversational user interfaces, or the chatbot virtual assistant, but its weaknesses, including lack of reliability, hallucinations and the illusion of thinking when really there is limited reasoning, can derail use cases.
“Nowadays with really well done generative AI applications that have great prompting you may get two percent failure rate,” Elliot says of the issue of hallucinations. One vendor has been claiming one percent failure, but Elliot notes for anyone doing something critical or doing a million prompts in production, even a one percent failure isn’t acceptable.
“There is a gap between what people are talking about [and want generative AI to do] and what it can actually deliver.
“Right now there are a lot of solutions out there that have not been thought through far enough and they may not end up in a useful result.”
Companies which solely focus on GenAI risk an increased failure in their AI projects, and risk missing out on many opportunities, he says.
Among the areas where generative AI is the wrong tool, according to Elliot, are
– Planning and optimisation,
– Predicting and forecasting,
– Decision intelligence, and
– Autonomous systems.
“Planning and optimisation scenarios such as marketing allocation, route optimisation and production planning are areas where GenAI is not going to succeed because large language models don’t carry out exact calculations and then plan out an answer,” Elliot says.
While there is a lot of work being done to try and address that issue of planning, he says for now, using GenAI for such a use case is likely to result in flawed responses for even simple planning and optimisation tasks.
“A better tool to use would be techniques like optimisation techniques, knowledge graph techniques or composite AI where several methods are used together to get to the right answer.”
When it comes to prediction and forecasting, such as customer churn prediction, sales and demand forecasting and predictive maintenance, generative is not good at the numerical and statistical modelling required.
Instead companies can use predictive machine learning algorithms or a stimulation or causal.
On the decision intelligence front, for example with claims approval for insurance, credit approval for finance and recruitment decisions in HR, GenAI struggles because it is not explainable.
“When it gives you an answer you can’t ask how it came up with that answer. You don’t know why the answer was achieved. And it’s unreliable and the risk of bias is a big one.”
Alternatives include rule-based systems, optimisation simulation and composite AI.
The final area Elliot called out was autonomous systems such as automated trading, self-driving cars and robotics.
“GenAI struggles here because of lack of reliability, and typically you want a human in the loop in these kinds of tasks and really good guardrails because these have significant ramifications if you make a mistake.”
While ‘agentic’ models, or agent-based solutions seem to be the push du jour for vendors, Elliot was wary, urging companies to beware of AI agent washing and ‘crack it open’.
“Agents are still emerging. Software AI agents are basically a subroutine that is encapsulated that you can call from anywhere so you still want to treat them like a piece of software.
“Ask what’s in there? What is the AI being used for? What are the decisions being made and is this something that is a good use case?
“Many vendors will be announcing AI agents, but few of them will live up to that name.”
Reinforcement training and optimisation are better options than Generative AI for autonomous systems, he says ‘but it really does depend on the kind of agent and the kind of autonomy it is being given’.
“GenAI is not the only AI in town,” Elliot says, urging companies to target the technology at the right problem – and harness other forms of AI for other areas.
“AI is a toolbox and GenAI is just one of the tools in that toolbox.”