Published on the 03/08/2023 | Written by Heather Wright
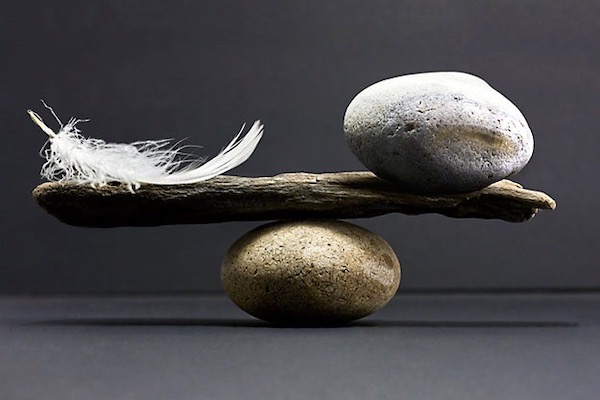
The pragmatic introduction of AI techniques…
Data science and machine learning is evolving from focusing on predictive models towards a more democratised, dynamic and data-centric discipline – and one fuelled by the fervour around generative AI, attendees at Gartner’s Data and Analytics Summit have heard.
While it might have been the analyst firm’s annual Sydney Data and Analytics conference, it was AI that was very much to the fore throughout and while AI might stand for artificial intelligence, several analysts were keen to stress that it’s more a case of ‘augmented intelligence’.
“Transformative projects should wait.”
Edge AI, responsible AI, data-centric AI, and the accelerated investment in AI were all held up as top trends shaping the future of data science and machine learning. (The only non-AI trend was data ecosystems moving from self-contained software or blended deployments to fully cloud-native solutions.)
A Gartner poll of more than 2,500 executive leaders found 45 percent have increased AI investments on the back of hype around ChatGPT.
Erick Brethenoux, who leads Gartner’s AI steering council, provided a reality check for companies caught up in the generative AI hype, as he reminded attendees that AI has been around for many years.
“The spotlight is so bright, there is no way we can actually achieve all the expectations. It’s the Arthur C Clarke saying all over again – any sufficiently advanced technology is indistinguishable from magic,” Brethenoux says, quoting from Clarke’s 1962 book, Profiles of the Future: An Inquiry into the Limits of the Possible.
“That is the problem we are facing today.”
He noted that AI, rather than being a singular technology, is a set of software engineering techniques that simulate – a word he was at pains to emphasise – cognitive processes.
“The computer does not understand anything. It stores and computes. That’s all it does. Then we anthropomorphise those capabilities by saying it learns. No, it doesn’t.
“We need to anthropomorphise to understand the system better. That’s ok, so long as we don’t slip into the computer understands or this or that.”
Among the techniques called on by AI are machine learning, including deep learning – which again Brethenoux was keen to stress was not ‘deep’ in the sense of intelligence, but merely in its ability to harness thousands of layers of information – rule-based systems, optimisation techniques, natural language processing, knowledge representation, including knowledge graphs, and agent based computing and orchestrating.
“Those are all techniques that have been around 30 years.”
For the record, ChatGPT is a practice of AI, born out of deep learning but also using machine learning techniques, NLP, rule-based systems and, increasingly, knowledge graph tech.
He urged attendees to step up and help educate people – including executive teams – and debunk many of the myths surrounding the technology, saying many are confused and have misconceptions.
“Educate, inform evangelise, tell what the systems do and don’t do, where they fail, where they succeed.
“Inform people, that is the best way. Managing expectation is hard especially these days after all the hype,” he says.
When it comes to introducing AI techniques to solve complex business problems, he noted several families of AI techniques often need to be used in sequence to solve a problem, and urged attendees to develop several – rather than just one – early use cases that address and improve challenges in the business now.
“Transformative projects should wait.”
Identifying who will work on the project – and not just AI experts but the subject matter experts who will help solve the problems and the software engineers who will eventually implement the solution within existing software and applications – forms the second prong.
Then comes the thorny issue of data.
“And when I say ‘do we have the data’ I do not mean do we have enough data. I mean do we have access to the data? Is it lawful? Are you violating any privacy to get to that data? Once we have the problem and the solution in production do we have access to that data? Is it clean enough? Coming fast enough, are there data pipelines in place for this?”
The technology component comes in at fourth on Brethenoux’s formula for AI techniques introduction.
“And as an engineer it hurts me to have tech in the fourth position. But it is there. Once we know what we are going to do, what people we have available to work on this, what data we have available at our disposal, then we can start to look at the techniques. Maybe we don’t have enough data for ML capability but graph can solve a problem in that case. And so on.”
After the process has been done a few times and developed several minimal viable products, he says companies can think about organisation, including where the AI team fits.
When it comes to trends, Brethenoux noted a big movement among Gartner clients from data-centricity to decision-centricity and looking at the outcome, rather than just the data.
“It’s not one or the other. We can do decision-centricity because we have data-centricity, so we build on top of it, we use a higher level view on top of it. But decision-centricity is becoming one of the main topics that I hear across my clients. It’s a big undercurrent that is irresistible moving forward.”
Perhaps controversially, Brethenoux urged businesses not to start with open source, and to instead look at the buy option.
“In AI you need to know what you are doing. It’s like getting a box from Ikea where you don’t have the instructions and some pieces are missing.”
In his final parting shot, he warned that the time to learn is incompressible and companies can’t afford to wait too long.
“Even if you do a small project, a small thing to get educated, that’s the way to go in my opinion.”
AI also featured in Gartner’s top trends for data and analytics, unveiled at the summit. Gareth Herschel, Gartner VP analyst, noted 10 trends ranging from value optimisation and managing AI risk to practical data fabric, emergent AI and converged and composable ecosystems.
Consumers becoming creators and humans remaining decision makers also featured.
“Not every decision can or should be automated,” Herschel says.
“Efforts to drive decision automation without considering the human role in decisions will result in a data-driven organisation without conscience or consistent purpose.”