Published on the 19/12/2024 | Written by Heather Wright
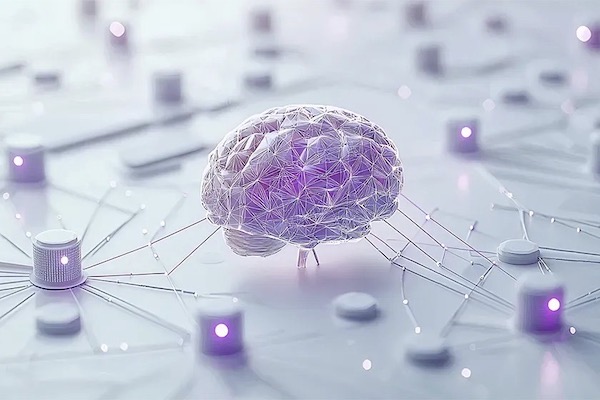
LLMs just the ‘bricks’ for a new future…
The large language models so hyped in the past two years since ChatGPT burst into popular consciousness are merely ‘bricks’ – it will be agentic AI which enables us to take those bricks and build the enterprises of the future.
That’s according to Leslie Joseph, a principal analyst with Forrester, who says despite all the hype we’re still in the very early stages of both AI and agentic AI.
“It moves the concept of what you can do with generative AI away from ‘hey, tell me stuff’ to ‘hey, do stuff for me’.”
Agentic AI has become the big topic in recent months, with vendors quick to pronounce that ‘the future is agentic’. They’re not on their own however, with the big analyst firms equally bolshy about the future of AI agents – Gartner has positioned agentic AI as one of the most transformative forces set to reshape enterprises in the coming 24 months, saying it will introduce a goal-driven workforce that autonomously makes plans and takes actions, becoming an extension of the workforce that doesn’t need vacations or other benefits.
Meanwhile, a recent Capgemini report said more than four out of five executives plan to integrate AI agents within the next three years.
SAP, Microsoft, Salesforce, ServiceNow are among the long list of companies touting agents. This month Google joined the fray introducing Agentspace, a service designed to create and deploy AI agents, providing a launch point for custom agents that apply generative AI contextually.
Joseph’s definition of agentic AI is a little more defined than that of some vendors jumping on the agentic bandwagon.
He defines agentic workflows as ‘AI systems designed to autonomously perform tasks or make decisions acting as independent agents in dynamic environments’.
Essentially, agents enable LLM models to combine with other models, talking to specialised agents and taking independent action. Where previous generations of AI systems focused largely on pattern recognition and predictive analytics, agentic AI adds the independence to execute workflows with minimal human involvement.
“It moves the concept of what you can do with a LLM or generative AI system away from ‘hey, tell me stuff’ to ‘hey, do stuff for me’.
He says agents are the coming together of six design patterns: Planning, reflection, multi-agent collaboration, tool use, memory and state and autonomy.
The agent can plan, which means it can take a task, break it down and do specific sub-components. It can reflect on that plan and refine it over time through specific frameworks available today. Multiple agents with ‘super specialisations’ can collaborate with each other, taking input from other agents – not in a deterministic coded manner but ‘just agents talking to other agents using natural language communication and doing things’, Joseph says.
Importantly, agentic AI also includes tool use. “This is the fact that an agent can do things in the real world by generating code or essentially function calling. It can use tools like APIs and microservices to create outputs so if you want to book a ticket, it can do that.”
While an LLM on its own doesn’t have a memory, agents will need to in order to know what has been completed and move onto the next thing on the list.
“When you start building you realise some of these agents you can create with fairly lightweight tools and even the skillsets you need to bring to bear don’t need to be advanced coding skillsets, you have frameworks out there like AutoGen and so on that allow you to create agents in a fairly simplistic way.”
Emerging use cases will be goal oriented. Accounts receivable agentic AI could detect delinquent status, generate custom letters from core systems and follow up.
Health agents could transcribe doctor’s notes, populate patient medical records and orchestrate sign-off while IT help desk agents could interpret employee problem statements, resolve technical issues and deflect tickets from the help desk. Financial assistants could detect action, retrieve correct forms, prefill forms from core systems and load the forms into a customer portal ready for signatures, while a sales agent could gather pipeline leads, make outbound calls, handle objections, collect prospect information and schedule meetings.
A Forrester heatmap of AI agent use cases shows financial services and insurance running ‘warm/hot’ when it comes to sales qualification and support, with internal operations another hotspot for insurance – and for smart manufacturing. For healthcare the hotspot is technical support while for the public sector, it’s employee self-service likely to lead the charge.
“There are hundreds of thousands of use cases that are yet to happen – remember, we have just invented the brick. Now we are going to start building the cities and palaces that are really going to transform the enterprise,” Joseph says.
He warns however, that agentic AI is not just reshaping the way work takes place, but also what the enterprise tech stack looks like in the future, as it moves from a portfolio of enterprise apps to a catalogue of skills such AI agents, machine learning models, microservices and process automations.
“We are starting to see it is not the application or interface or what have you, but the skill that is almost like a lego block that gets configured and reconfigured with other lego blocks to deliver an outcome and respond to different things happening in the environment. That is going to be a very big change in the way we think about the enterprise tech stack.”
If it all sounds a little ‘pie in the sky’ Joseph is adamant it is not, saying agents are already making their way into the enterprise.
“We already see enterprises starting to look at specific business processes within the enterprise and say how can we make this more autonomous? They are injecting agentic behaviour and agentic applications in to this so a lot of the things you used to need humans for, or things that needed multilevel interactions with humans and chains of approvals and so on, are now being rapidly handled and managed and orchestrated by agentic tools and capabilities.”
But despite his positivity about agentic AI, Forrester is also sounding a warning, predicting that three out of four firms that build aspirational agentic architectures on their own will fail.
And what will lead to those failures?
Having too broad, or too narrow, an vision in terms of the definition provided for the agents and the tasks they do and a poorly defined focus on specific use cases, which in turn will be related to either over- or underestimation of the capabilities of the agents and the context of the use cases, are among the key reasons.
A third reason, Joseph says, lies in the current largely DIY nature of the tooling around agents.
“The ecosystem is very DIY. That means there is a lot of very specific need for very highly skilled technical talents to build realistic and effective agentic architectures. So the technical solution itself might elude some organisations.”
Also likely to set a company up for failure is being too focused on the agentic component, ignoring the fact that agents sit on processes and those processes need to be orchestrated and automated in a specific way to drive the right outcomes.
Rounding out Joseph’s list of things to avoid if you don’t want to be one of the three in four failures: The agentic AI versus human disconnect, with change management and cultural alignment big stumbling blocks for companies as they start to adopt and bring agents deeper into their workforce and workflow.
“There is no easy button. You need to prepare for the grind.”