Published on the 28/06/2019 | Written by Heather Wright
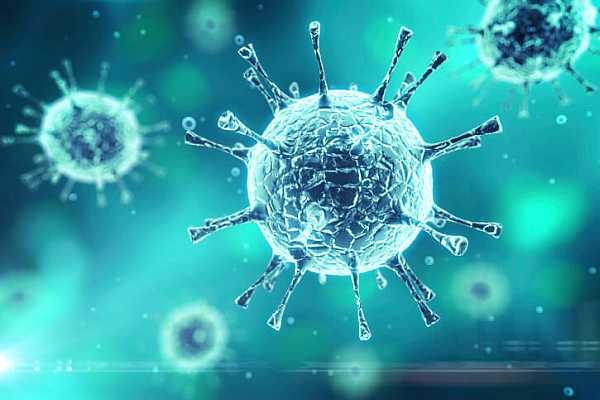
‘Vaccine’ to protect algorithms against attack…
Data61, the data and digital specialist arm of Australian national science agency, CSIRO, has taken a leaf out of medical textbooks to develop a ‘vaccine’ to protect AI and machine learning algorithms against attacks.
Despite their smarts, AI and machine learning are apparently easily confused and vulnerable to adversarial attacks which can fool machine learning models.
Richard Nock, Data61’s machine learning group leader, cites a computer vision example of attackers adding a layer of noise – an adversary – over an image, to deceive machine learning models into misclassifying an image. Images obvious to the human eye are misinterpreted by the slightly distorted image created by the attacker.
“When the algorithm is trained on data exposed to a small dose of distortion, the resulting model is more robust.”
“Adversarial attacks have proven capable of tricking a machine learning model into incorrectly labelling a traffic stop sign as a speed sign, which could have disastrous effects in the real world,” Nock says.
Google has previously noted the use of adversarial noise to trick machine learning modelling into thinking an image of a panda was actually a gibbon and an image of a temple was actually an ostrich.
“When it comes to deploying machine learning in safety-critical contexts, significant challenges remain,” Google says. “While previous research on adversarial examples has mostly focused on investigating mistakes caused by small modifications in order to develop improved models, real-world adversarial agents are often not subject to the small modification constraint.”
Similar pattern overlays can also be used with speech, tricking machine learning models into interpreting it incorrectly.
The programming technique developed by Data61 works on the same principle as vaccinations, providing a weak version of an adversary, such as small modifications or distortion to a collection of images to create a more ‘difficult’ training data set.
“When the algorithm is trained on data exposed to a small dose of distortion, the resulting model is more robust and immune to adversarial attacks,” Nock says.
CISRO says as the vaccination techniques are built from ‘the worst possible adversarial examples’ they’re able to withstand ‘very strong attacks’.
Adversarial attacks on machine learning have been gaining.
US researchers recently highlighted the dangers of adversarial attacks on medical machine learning systems, citing one example of adversarial noise being used to get algorithms to diagnose benign moles as malignant with 100 percent confidence.
Data61 presented its research paper, Monge blunts Bayes: Hardness Results for Adversarial Training at the 2019 International Conference on Machine Learning (ICML) earlier this month and Data61 CEO Adrian Turner says the new techniques will spark a new line of machine learning research.
“Artificial intelligence and machine learning can help solve some of the world’s greatest social, economic and environmental challenges, but that can’t happen without focused research into these technologies,” Turner says.
The organisation has been a key figure in the push for the ethical use of AI, and led the development of an AI ethics framework for Australia, which was released by the Government for public consultation in April.
“As AI becomes more integrated into many aspects of our lives, ‘vaccinations’ such as ours, are essential to the progression of a protected and safe innovative future,” CISRO says.
The ‘vaccine’ option has yet to be tested in real-world situations against genuine malicious attempts.