Published on the 17/12/2019 | Written by Rita Sallam
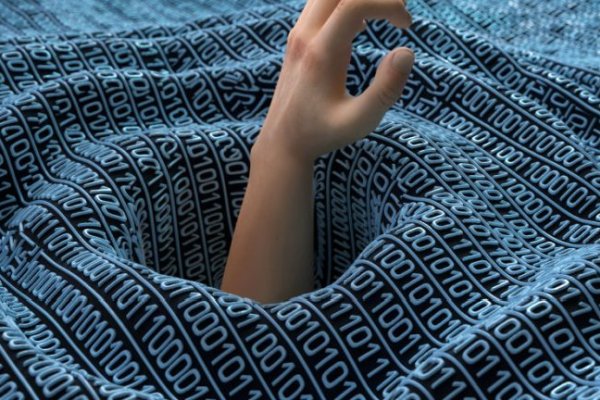
Analytics is at an inflection point…
Business people are drowning in data, struggling to identify what’s most important and what best actions to take. Larger and more varied dataset combinations also mean more variables and relationships to analyse, explore and test.
Across the analytics stack, tools have become easier to use and more agile, enabling greater access and self-service.
Many processes, however, remain largely manual and prone to bias. These include managing data, preparing data for analysis, analysing data, building data science and machine learning (ML)/artificial intelligence (AI) models, interpreting results, telling stories with data and making insights actionable.
Augmented analytics helps find the needle faster by acting like a giant magnet hovering over the hay.
Using current approaches, it’s not possible for users to explore every possible combination and pattern, let alone determine whether their findings are the most relevant, significant and actionable.
Relying on business users to find patterns and data scientists to build and manage models manually may result in them exploring their own biased hypotheses, missing key findings and drawing their own incorrect or incomplete conclusions. This will adversely affect decisions, actions and outcomes.
Introducing augmented analytics
To address these growing challenges, augmented analytics is rapidly gaining traction. Central to this development is the use of ML automation/AI techniques to augment human intelligence and contextual awareness. It is transforming data management, analytics and BI, as well as many aspects of data science and ML/AI model development and consumption.
Gartner predicts that by 2021, augmented analytics will be a dominant driver of new purchases of analytics and BI, as well as data science and ML platforms, and of embedded analytics.
As businesses become inundated with data, augmented analytics becomes crucial for presenting to users across the business only what’s important for them, in their context, to act upon at that moment.
Having to manually find patterns in the data is like looking for a needle in a haystack. Augmented analytics helps find the needle faster by acting like a giant magnet hovering over the hay.
Applying a range of algorithms to data in parallel, and explaining actionable findings to users, reduces the risk of missing important insights in the data in comparison to manual exploration.
It also optimises resulting decisions and actions. This shift requires investment in data literacy throughout organisations, as insights are distributed to all employees. As augmented analytics makes actionable insights available to users, it must also provide context, interpret findings and recommend how best to act on the discoveries. This requires everyone in the organisation — not just managers — to have some knowledge of data analysis, statistics and interpretation.
Addressing the skills gap
As more organisations digitally transform, they want to expand the use of data science and ML/AI. In particular, they want to use it to create new differentiated analytic applications and embed ML/AI into existing applications. The scarcity of expert data science skills has become a significant barrier.
By automating many time consuming and bias prone tasks for creating data science and ML models and AI, augmented analytics expands the capabilities of those with more widely available skill sets – the business analyst and the application developer (a new breed of citizen data scientists). In collaboration with expert data scientists, it allows them to generate insights and create augmented analytics-assisted models to embed in applications.
Since augmented analytics automates feature engineering, model selection and increasingly model management and model operationalisation (MLops) tasks, augmented analytics can also make expert data scientists more productive.
Rapidly advancing to mainstream
Due to the perception of high potential impact, augmented analytics capabilities will advance rapidly to mainstream adoption.
More importantly, automated insights from augmented analytics is also being embedded in enterprise applications, natural language processing (NLP)-enabled applications and chatbots for analytics to make insights available to more people across the organisation.
More than 60 percent of respondents to a Gartner Data and Analytics Summit poll said they believe augmented analytics will have a high or transformational impact on their ability to scale the value of analytics in their organisation.
Innovative startups and large vendors now offering augmented analytics capabilities are transforming how people generate and consume analytics insights. They’re disrupting data and analytics markets, spanning analytics and BI, data science and ML platforms, as well as analytics embedded in enterprise applications.
Given the business impact and market potential, remaining vendors will quickly follow, and adoption will accelerate quickly as the technology matures.
Demonstrate potential value
Efforts to incorporate augmented analytics will likely encounter resistance. There’s a perception that augmented analytics tools aren’t transparent and represent a ‘black box’ approach to decision-making. This can be mitigated with explainability features that are increasing available with augmented analytics.
The threat to job security, as redivision of workloads and redefinition of work processes, also upsets the status quo and should be addressed.
An effective way to demonstrate the potential value of augmented analytics is to identify business problems or specific business decisions where efforts to use current data and analytics approaches have failed to deliver results in timely, relevant or actionable ways. Back test on historical data to build trust. By doing so, you can lay the foundation for new, more automated approaches.
ABOUT RITA SALLAM//
Rita Sallam is a distinguished VP analyst at Gartner in the data and analytics team. She focuses on tracking and predicting data and analytics market trends, vendor assessment and selection, and identifying best practices for making analytics pervasive and strategic to the business.